Introduction
How does data-driven attribution work?
When figuring out how your various ads contribute to customer actions, data-driven attribution analyzes the customer journey to assign credit to different touchpoints. It uses machine learning to understand the impact of each interaction, providing a detailed view of which ads drive conversions.
Data-driven attribution helps marketers:1. Identify key ad interactions that lead to conversions.2. Optimize bidding strategies based on which ads are most effective.3. Allocate budget more effectively by understanding the role of each ad.
In today's digital landscape, knowing where and how your marketing efforts pay off is crucial. This is where data-driven attribution comes in. It goes beyond traditional models like last-click attribution to give you a clearer, more precise picture of what's driving your business goals.
Over time, digital marketing has become incredibly complex. Customers interact with brands through multiple platforms and devices before making a purchase. Ignoring these interactions can lead to incomplete insights and missed opportunities. Data-driven attribution solves this by analyzing all these touchpoints to determine their actual contribution to your conversions.
By understanding the full customer journey, you can fine-tune your marketing strategies. This helps in making smarter decisions and maximizing your ROI. And as technology advances, this method of attribution will keep evolving, offering even more accurate insights for future growth.
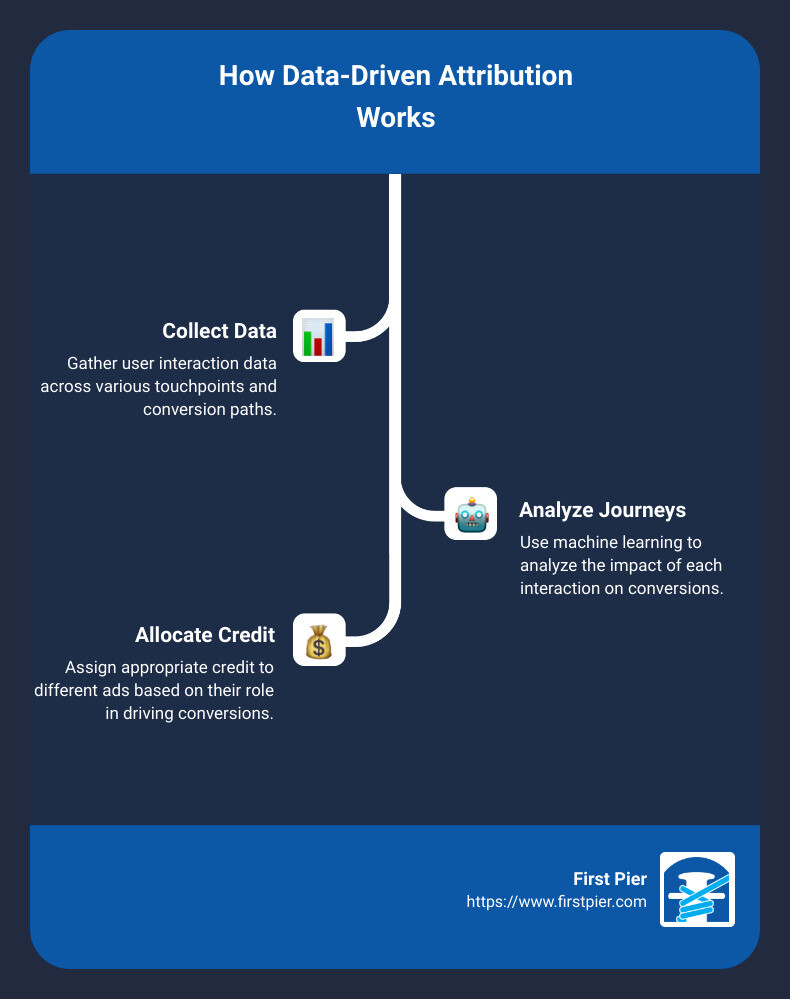
What is Data Driven Attribution?
Data-driven attribution is a method that assigns credit for conversions based on how users interact with your various ads and decide to become customers. Unlike traditional models that might give all the credit to the last-clicked ad, data-driven attribution analyzes the entire journey a user takes before converting. This includes every click, view, and interaction across different ads and channels.
Mechanics of Data Driven Attribution
Data-driven attribution uses historical data and machine learning algorithms to evaluate the impact of each touchpoint in the customer journey. Here's how it works:
- Data Collection: The system collects data from all interactions users have with your ads. This includes clicks, video views, and other engagements across Search, YouTube, Display, and Demand Gen ads.
- Path Analysis: The model compares the paths of users who convert with those who don't. By identifying patterns, it can determine which interactions are more likely to lead to a conversion.
- Credit Allocation: Based on the analysis, the model assigns more credit to interactions that have a higher probability of leading to a conversion. This helps in understanding which ads, keywords, and campaigns are most effective.
For example, imagine you run a tour company in New York City. If a customer first clicks on a "Bike tour New York" ad and later clicks on a "Bike tour Brooklyn waterfront" ad before purchasing a ticket, the model might find that the first ad played a crucial role in the decision-making process. It would then allocate more credit to the "Bike tour New York" ad.
Importance of Data Driven Attribution
Understanding the full customer journey is crucial for several reasons:
- Optimized Marketing Spend: Knowing which ads and keywords contribute most to conversions allows you to allocate your budget more effectively.
- Improved ROI: By focusing on the touchpoints that matter most, you can enhance your return on investment.
- Better Decision-Making: Data-driven insights help you make more informed decisions, improving your overall marketing strategy.
According to Think with Google, 76% of marketers say they either have or will have the capability to use marketing attribution within the next 12 months. This shows the growing importance of understanding which marketing efforts drive results.
Pro Tip: Tools like Ruler Analytics use data-driven attribution to model the customer journey and assign credit to different touchpoints. This can be incredibly useful for businesses looking to optimize their marketing strategies.
In the next section, we'll dive into how data-driven attribution works specifically in Google Ads, exploring the role of machine learning and conversion paths.
How Does Data Driven Attribution Work in Google Ads?
Understanding Google's Data-Driven Model
Google's data-driven attribution model is a game-changer for marketers. Unlike traditional models that give all the credit to the last ad clicked, this model uses machine learning to analyze the entire customer journey. This means it looks at all the interactions—clicks, video views, and more—across various Google ad platforms like Search, YouTube, and Display.
By comparing the paths of customers who convert to those who don't, Google's model identifies patterns and assigns more credit to interactions that are more likely to lead to a conversion. For example, if a customer clicks on your "Bike tour New York" ad first and then clicks on "Bike tour Brooklyn waterfront" before making a purchase, the model will give more credit to the first ad if it finds that this sequence often leads to conversions.
Historical Data plays a significant role here. The model analyzes past interactions and conversions to understand what works best. This way, you get a tailored model that reflects your specific business dynamics.
Benefits of Using Data-Driven Attribution in Google Ads
Improved ROI
One of the most compelling benefits of data-driven attribution is its ability to improve your Return on Investment (ROI). By understanding which ads truly contribute to conversions, you can allocate your budget more effectively. According to Google, combining this model with automated bidding strategies can drive additional conversions at the same cost-per-acquisition.
Accurate Credit Allocation
With data-driven attribution, you get accurate credit allocation across all touchpoints. No more guessing which ad made the difference. The model's machine learning algorithms ensure that each interaction is evaluated based on its actual contribution to the conversion. This means you can finally see the real value of your top-of-the-funnel and middle-of-the-funnel ads.
Enhanced Bidding Strategies
Another significant advantage is the enhancement of your bidding strategies. Since the model provides a clearer picture of which ads are driving conversions, automated bid strategies can use this information to optimize bids. This helps you get the most out of your ad spend, ensuring that your bids are placed where they are most likely to convert.
Example: Imagine you're running a campaign for a new product. With last-click attribution, you might think that only the final ad interaction was crucial. However, with data-driven attribution, you might discover that an earlier display ad played a significant role in the customer's journey. This insight allows you to adjust your bids and ad placements to maximize effectiveness.
Pro Tip: Start by setting up data-driven attribution in your Google Ads account. Navigate to the Attribution model settings and select "Data-driven attribution" from the drop-down menu. This will enable the model to start analyzing your data and providing actionable insights.
In the next section, we'll explore how data-driven attribution works in Google Analytics, focusing on user journeys and touchpoints.
How Does Data Driven Attribution Work in Google Analytics?
Data-driven attribution in Google Analytics 4 (GA4) uses machine learning and statistical algorithms to analyze user journeys and touchpoints across your digital properties. This approach provides a more nuanced understanding of how different marketing channels contribute to conversions.
Key Features of Data-Driven Attribution in GA4
1. Machine Learning and Statistical AlgorithmsGA4's data-driven attribution relies on advanced machine learning models to evaluate the impact of each touchpoint in the customer journey. These models analyze historical data to identify patterns and assign credit to the interactions that most likely led to conversions.
2. Cross-Platform IntegrationOne of the standout features of GA4 is its ability to integrate data from various Google products, including Google Ads, Google Display, and Campaign Manager 360. This holistic view allows you to see how different channels work together to drive conversions.
3. Comprehensive User JourneysGA4 tracks user interactions across multiple sessions and devices, providing a complete picture of the customer journey. This includes everything from the first site visit to the final conversion, allowing you to understand the role of each touchpoint.
4. Customizable Look-Back WindowsGA4 allows you to set different look-back windows for various conversion events. For instance, you can choose a 30-day window for acquisition events and a 90-day window for other conversions. This flexibility helps you tailor the attribution model to your specific business needs.
Setting Up Data-Driven Attribution in Google Analytics
Configuration Steps
- Sign in to Google Analytics 4: Navigate to the property you want to set up data-driven attribution for and click on Admin.
- Navigate to Attribution Settings: In the Admin panel, find and click on Attribution Settings.
- Select Data-Driven Attribution: By default, data-driven attribution should be selected. If not, you can choose it from the drop-down menu.
- Set Look-Back Windows: Scroll down to set your look-back windows for different conversion events. This step is crucial for tailoring the model to your specific needs.
- Save Settings: Click Save to apply your settings.
Eligibility Requirements
To use data-driven attribution in GA4, your account needs to meet certain data requirements. Specifically, you must have a sufficient amount of conversion data and touchpoint data. This ensures the machine learning models have enough information to provide accurate insights.
Data Analysis
Once set up, GA4 will start analyzing your data to identify the most impactful touchpoints. You can access these insights through various reports, such as the Conversion Paths and Model Comparison reports. These tools allow you to see how different channels contribute to conversions and compare the effectiveness of various attribution models.
Overall, data-driven attribution in GA4 provides a more accurate and comprehensive view of your marketing efforts, enabling you to make data-driven decisions that optimize your campaigns.
In the next section, we'll compare different attribution models to help you understand the benefits and limitations of each.
Comparing Attribution Models
Transitioning to Data-Driven Attribution
When moving from traditional attribution models to data-driven attribution (DDA), understand the differences between these models. Here's a quick overview of some common attribution models and how data-driven attribution stands out.
Last Click
Last Click attribution gives all credit to the final touchpoint before the conversion. This model is simple but often misleading, as it ignores the influence of earlier interactions.
Example: If a customer clicks on a Google ad and then makes a purchase, the last-click model attributes the entire conversion to that ad, even if the customer first discovered your brand through a social media post.
First Click
First Click attribution assigns all credit to the first touchpoint. This model is useful for understanding initial brand awareness but doesn't account for the role of subsequent interactions.
Example: If a customer first clicks on a blog post and later makes a purchase after several other interactions, the first-click model gives all credit to the blog post, ignoring the other touchpoints.
Linear
Linear attribution distributes credit evenly across all touchpoints in the customer journey. While more balanced, it assumes each touchpoint is equally important, which may not always be the case.
Example: If a customer interacts with your brand through an email, a social media post, and a Google ad before purchasing, the linear model gives equal credit to all three interactions.
Time Decay
Time Decay attribution gives more credit to interactions that occur closer to the conversion. This model is based on the idea that recent touchpoints are more influential.
Example: If a customer clicks on an ad, then reads an email, and finally makes a purchase after seeing a retargeting ad, the time decay model gives more credit to the retargeting ad.
Position-Based
Position-Based attribution, also known as the U-shaped model, gives 40% credit to the first and last interactions and distributes the remaining 20% among the middle interactions. This model recognizes the importance of both initial awareness and final decision-making.
Example: If a customer discovers your brand through a search ad, engages with several social media posts, and finally converts via an email, the position-based model gives significant credit to both the search ad and the email.
Simulation Tools
Before fully transitioning to a data-driven attribution model, it's wise to use simulation tools to compare performance across different models. Google Ads and Google Analytics offer Model Comparison tools that allow you to see how conversions and credit allocation differ under various models.
Example: By comparing the last-click model with the data-driven model, you might discover that certain keywords or channels play a more significant role in conversions than previously thought.
Performance Impact
Switching to a data-driven attribution model can have a substantial impact on your marketing performance. According to Google, advertisers using DDA have seen a 6% increase in conversions without increasing budget. This is because DDA provides a more accurate picture of how different touchpoints contribute to conversions.
Model Comparison
When comparing different attribution models, consider the following:
- Accuracy: Data-driven attribution uses machine learning to analyze both converting and non-converting paths, providing a more accurate representation of the customer journey.
- Flexibility: Unlike heuristic models, DDA adapts to changes in user behavior and marketing strategies.
- Insights: By understanding the true impact of each touchpoint, you can optimize your marketing efforts for better ROI.
Table: Attribution Model Comparison
ModelCredit AllocationBest ForLast ClickFinal touchpoint onlySimple, easy to implementFirst ClickInitial touchpoint onlyInitial brand awarenessLinearEven distribution across all touchpointsBalanced viewTime DecayMore credit to recent touchpointsRecency importancePosition-Based40% to first and last, 20% to middle touchpointsInitial and final interactionsData-DrivenBased on historical data and impactAccurate, adaptive, insightful
In the next section, we'll dive into frequently asked questions about data-driven attribution to clear up any lingering doubts and help you leverage this powerful tool effectively.
Frequently Asked Questions about Data Driven Attribution
How does data-driven attribution differ from other models?
Data-driven attribution (DDA) stands out because it analyzes both converting and non-converting paths. Unlike rule-based models like last-click or first-click, DDA uses historical data to understand the impact of each ad interaction on conversions.
Heuristic models:- Last Click: Credits the last interaction.- First Click: Credits the first interaction.- Linear: Distributes credit evenly across all touchpoints.- Time Decay: Gives more credit to interactions closer to the conversion.- Position-Based: Allocates 40% to the first and last interactions, and 20% to the middle.
Data-Driven Attribution:- Uses machine learning to analyze data.- Considers every interaction in the customer journey.- Adapts to changes in user behavior and ad performance.
For example, if removing an ad interaction drops the conversion rate significantly, DDA will assign higher credit to that interaction. This method is more accurate and insightful.
What are the prerequisites for using data-driven attribution?
To use data-driven attribution effectively, you need:
- Sufficient Data: Most conversion actions are eligible, but having a robust amount of interaction and conversion data improves accuracy.
- Google Analytics 4 (GA4): Ensure you’re using GA4, as Universal Analytics will no longer be supported after July 2023. GA4 offers advanced attribution features.
- Conversion Tracking: Set up conversion tracking to monitor actions like purchases, sign-ups, or downloads.
- Integration with Google Ads: Link your Google Ads account to GA4 for comprehensive data analysis.
Steps to Set Up DDA in GA4:1. Navigate to Advertising Snapshot in GA4.2. Go to Tools and Settings > Attribution > Model Comparison.3. Configure your DDA model and apply it to your reports.
How can data-driven attribution improve marketing strategies?
Data-driven attribution helps you optimize your marketing efforts by providing:
- Accurate Credit Allocation: Understand the real impact of each ad interaction, allowing for better budget allocation.
- Enhanced Bidding Strategies: Use insights from DDA to adjust your bids and maximize ROI.
- Improved ROI: By identifying and investing in the most effective ads, you can improve your return on investment.
Case Study:Imagine you run a tour company in New York City. DDA reveals that customers who first click on your "Bike tour New York" ad and then on "Bike tour Brooklyn waterfront" are more likely to purchase. This insight helps you allocate more budget to the "Bike tour New York" ad, driving higher conversions.
Real-World Example:Ruler Analytics uses the Markov chain model to track and value marketing touchpoints. By analyzing state transitions in the customer journey, they assign credit based on the impact of each touchpoint, leading to more informed marketing decisions.
In summary, data-driven attribution offers a nuanced and accurate view of your marketing efforts, helping you make data-driven decisions that enhance your overall strategy.
In the next section, we'll dive into frequently asked questions about data-driven attribution to clear up any lingering doubts and help you leverage this powerful tool effectively.
Conclusion
Future Trends
The future of data-driven attribution is promising. As machine learning and AI continue to evolve, these models will become even more sophisticated. We can expect:
- Enhanced predictive capabilities: Future models will predict not just what worked, but what will work, making proactive adjustments to campaigns.
- Cross-device and cross-platform integration: With the rise of multi-device usage, data-driven attribution will seamlessly track user journeys across all platforms.
- Privacy-focused solutions: As privacy regulations tighten, attribution models will adapt to provide accurate insights without compromising user privacy.
These advancements will make data-driven attribution an indispensable tool for marketers.
Decision Making
Using data to drive decisions is no longer optional; it's a necessity. With data-driven attribution, we can:
- Allocate budgets more effectively: Understand which channels and campaigns yield the highest ROI.
- Optimize marketing strategies: Identify and focus on high-performing touchpoints.
- Improve customer experiences: Tailor content and offers based on user behavior patterns.
By leveraging these insights, businesses can make more informed decisions, leading to better results and higher efficiency.
First Pier's Expertise in E-commerce
At First Pier, we specialize in helping businesses navigate the complexities of digital marketing, particularly in the realm of e-commerce. Our team of experts can guide you through the intricacies of data-driven attribution, ensuring you get the most out of your marketing efforts.
Whether you're new to e-commerce or looking to optimize your existing strategies, we provide tailored solutions to meet your unique needs. Our services include:
- SEO: Improve your search engine rankings and drive organic traffic.
- Content Creation: Develop engaging content that resonates with your audience.
- Social Media Marketing: Utilize social platforms to boost brand awareness and engagement.
If you're ready to take your online marketing to the next level, get in touch with us today. Let us help you unlock the full potential of your e-commerce business with a tailored data-driven attribution strategy.
Enjoyed the read? There’s a heap more where that came from! Hit the ‘Subscribe’ button below, it’s a two-second affair, but the bounty of e-commerce wisdom we share is endless. You’d be silly not to!